Is it possible to deploy a data project, from scoping to large-scale deployment, in 10 weeks? Let’s take a closer look at the keys to accelerating this type of project, which can sometimes take up to 18 months to generate value.
Before starting anything, it is essential to know fairly quickly whether there is a real need, a problem to be addressed, that could be addressed by such a project. But be careful not to fall into the traps of the “quick wins” approach. It is obviously tempting to produce quick results on a limited perimeter and thus make your stakeholders dream with the aim of obtaining support and funding. Nevertheless, there are at least two pitfalls in this approach:
- Pitfall 1: obtaining results that do not interest your business teams
Let’s take the example of a predictive model. The results of your project predict something that your business teams didn’t care about, or that doesn’t allow them to act. For example, your model predicts that the customer is about to leave but you have no leverage (no loyalty card or ability to send a coupon).
- Pitfall 2: “quick wins” should not mean a tiny data set
Predictive models require enough data to search for patterns. Rather than reducing the amount of data, it is often better to reduce the scope of the prediction.
Put the business vision at the heart of your project
Put the business vision at the heart of your project
In our column dedicated to the success of your Big Data / AI projects, we talked about the importance of involving the business, and this since the project was launched.
Remember that the business, the Data Lab and the IT teams are the key players in your project and their involvement in the project is crucial for its future adoption. If the presence of IT and the Data Lab seems obvious, the presence of the business should be more obvious. In addition to bringing the business vision to the project, they are often the end customer of the project, and therefore the ones who will be the most likely to derive value from it.
Example of a business framing technology
Once you have clarified the vision and identified the necessary actors, you can move on to the next step: refining the need. Business scoping is generally done in three distinct steps. Each phase is marked by a workshop bringing together the key players.
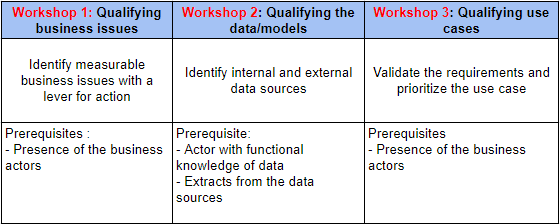
At the end of this step :
- you will confirm the presence of a significant signal in the data.
- you will have a first benchmark for your subsequent models.
The choice of big data infrastructure
After exploring your data, it’s time to choose the infrastructure to deploy your project. The functional and technical framework is also planned in three steps:
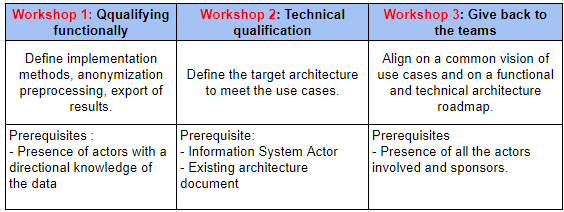
Most of the time you will have the choice between the “build” and “buy” approach.
- The “build” approach consists in designing and implementing the architecture created by the IT/Data architects in-house. You will thus keep control over the architecture deployed and the technologies chosen. However, the implementation of this architecture internally is often much longer than using external service providers (on average eighteen months).
- The “buy” approach is a turnkey solution that enables the project to start quickly and orchestrate its development and large-scale deployment from start to finish. This approach facilitates collaboration between IT, data and business teams in an open and shared environment. The major risk is to lock you into a specific technology that you or your teams don’t have control over.
At Saagie, we have chosen to offer you a ready-to-use DataOps solution orchestrating the best of Big Data technologies to accelerate the implementation and deployment of analytical projects. It is an agile and open solution integrating the widest choice of open-source technologies on the market and offering the level of security and governance necessary for the smooth running of your initiatives.
We believe that the keys mentioned in this article will serve as a guide to start your project in the best conditions.